Unlocking the Power of Predictive Analytics with Google Analytics Data
In the fast-paced digital landscape, staying ahead of the curve is essential for businesses. Predictive analytics with Google Analytics data, offers a powerful tool to anticipate customer behavior, optimize marketing efforts, and drive business growth.
This blog delves into the benefits, challenges, and the transformative role of data in predictive marketing. Additionally, we’ll explore deeper insights into predictive analytics techniques, practical implementation, emerging trends, and actionable takeaways.
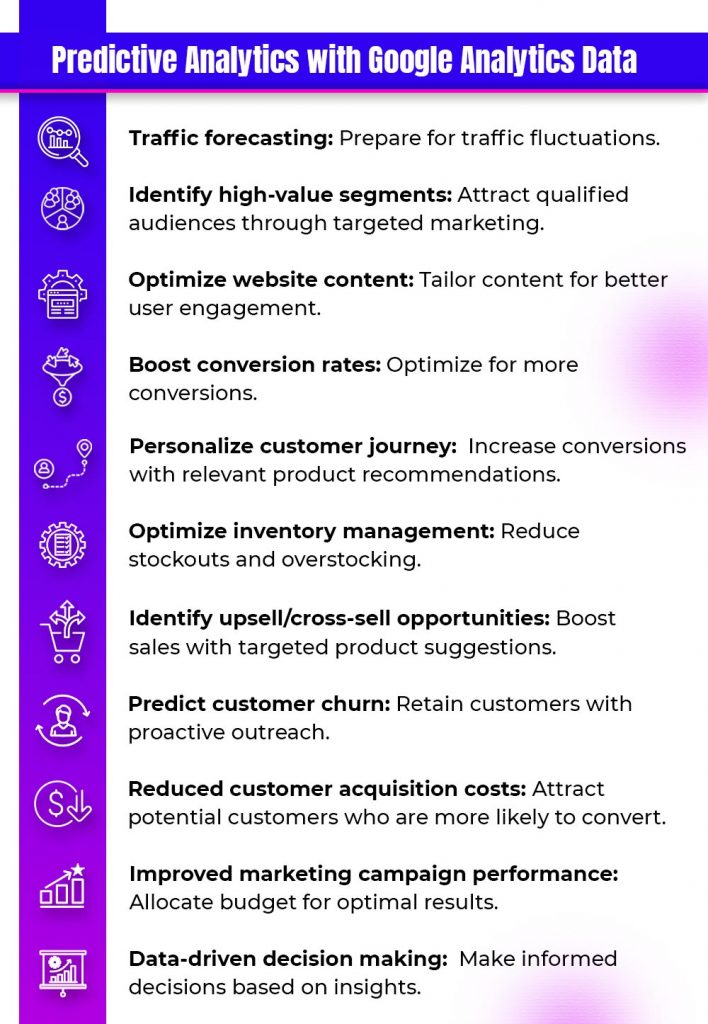
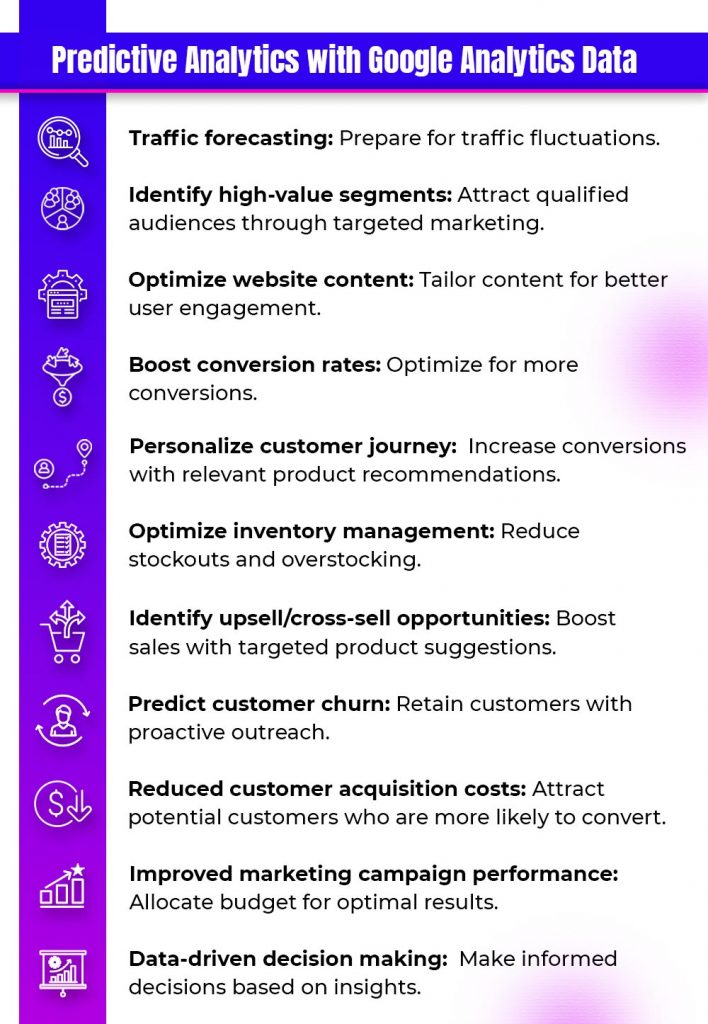
Benefits of Predictive Analytics with Google Analytics Data
Traffic Forecasting
One of the key advantages of predictive analytics is the ability to forecast website traffic. By analyzing historical data, businesses can predict traffic fluctuations and prepare accordingly. This foresight allows for better resource allocation, ensuring that websites can handle peak traffic periods without compromising on user experience.
Identify High-Value Segments
Predictive analytics helps in identifying high-value customer segments. By analyzing user behavior and engagement metrics, businesses can attract qualified audiences through targeted marketing campaigns. This not only increases the efficiency of marketing efforts but also enhances the return on investment (ROI).
Optimize Website Content
Tailoring website content to meet the preferences of your audience is crucial for user engagement. Predictive analytics provides insights into what type of content resonates best with users. This allows businesses to create and optimize content that keeps visitors engaged and encourages them to take desired actions.
Boost Conversion Rates
Optimizing for conversions is a top priority for any business. Predictive analytics enables businesses to understand the factors that drive conversions. By making data-driven adjustments to websites and marketing campaigns, businesses can significantly boost their conversion rates.
Personalize Customer Journey
Personalization is key to improving the customer journey. Predictive analytics helps businesses make relevant product recommendations based on user behavior and preferences. This personalization increases the likelihood of conversions and enhances the overall customer experience.
Optimize Inventory Management
For businesses dealing with physical products, inventory management is critical. Predictive analytics can forecast demand and optimize inventory levels, reducing the risk of stockouts and overstocking. This leads to more efficient operations and better customer satisfaction.
Identify Upsell/Cross-Sell Opportunities
Predictive analytics identifies opportunities for upselling and cross-selling by analyzing customer purchase history and behavior. By offering targeted product suggestions, businesses can increase their sales and average order value.
Predict Customer Churn
Retaining existing customers is often more cost-effective than acquiring new ones. Predictive analytics helps businesses identify customers who are at risk of churning. Proactive outreach strategies can then be implemented to retain these customers and maintain a loyal customer base.
Reduce Customer Acquisition Costs
By attracting potential customers who are more likely to convert, predictive analytics helps in reducing customer acquisition costs. Targeted marketing efforts ensure that resources are spent efficiently, leading to better ROI.
Improve Marketing Campaign Performance
Predictive analytics enables businesses to allocate their marketing budgets more effectively. By identifying the most promising channels and strategies, businesses can achieve optimal results from their marketing campaigns.
Data-Driven Decision Making
At its core, predictive analytics empowers businesses to make informed decisions based on actionable insights. By leveraging data, businesses can drive growth and stay competitive in the ever-evolving market.
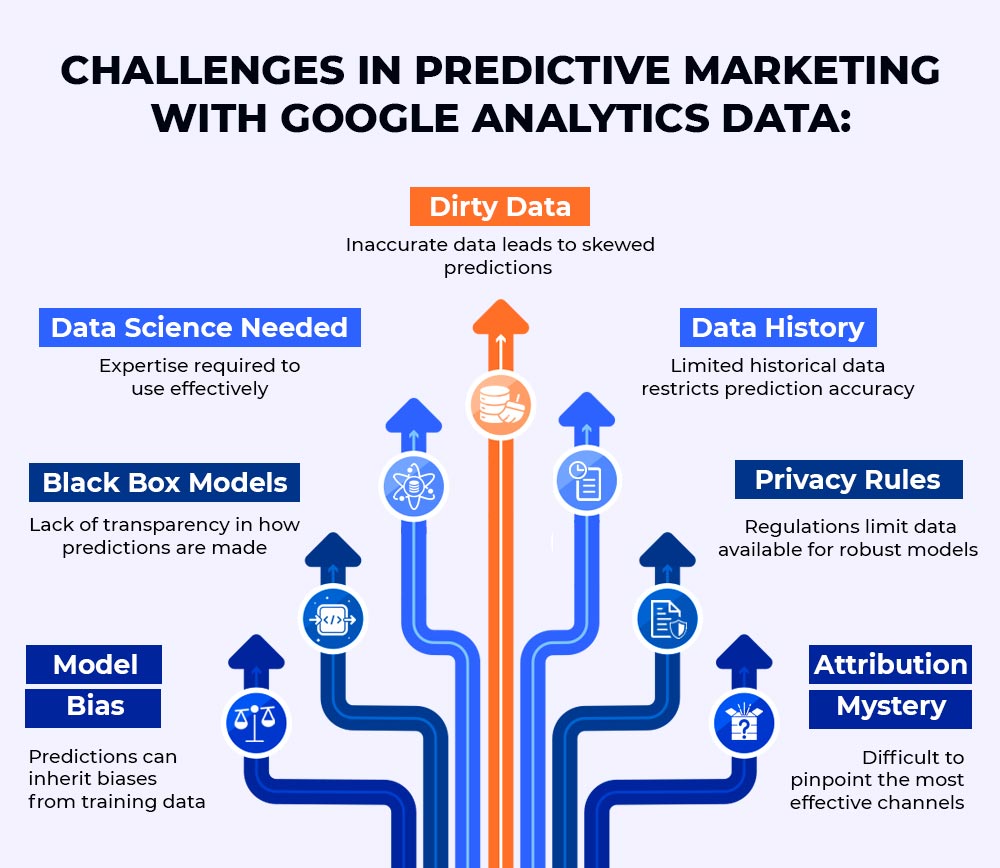
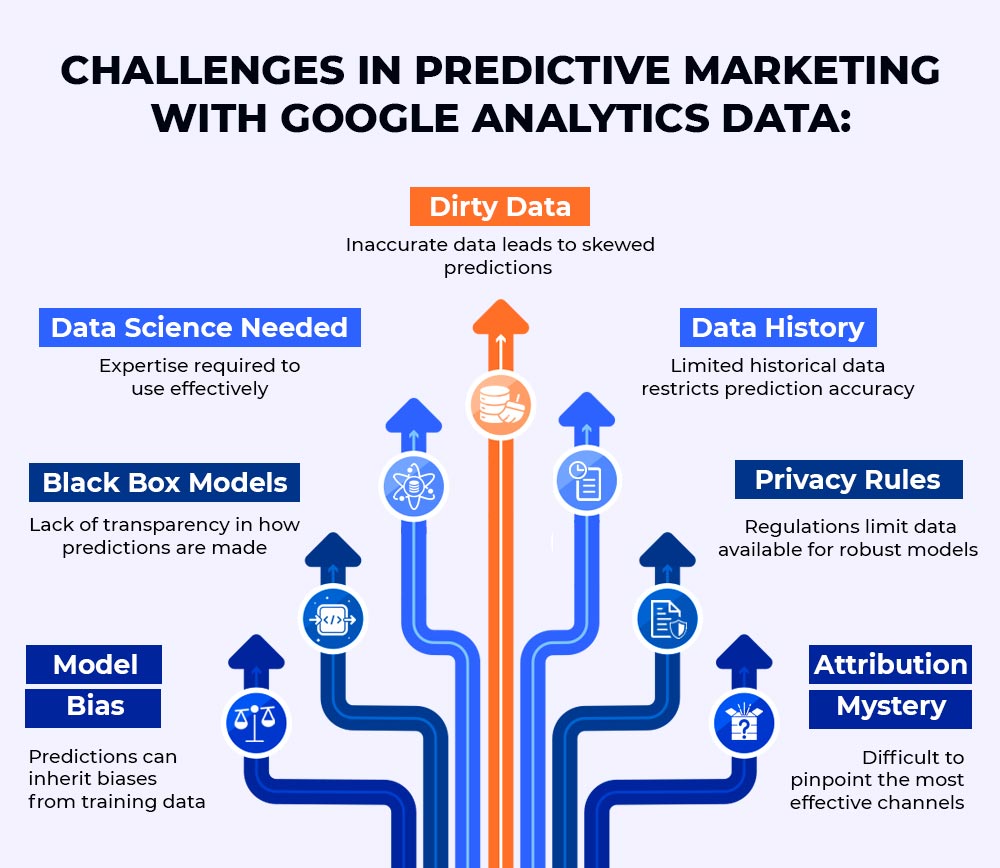
Challenges in Predictive Marketing with Google Analytics Data
Dirty Data
The accuracy of predictions heavily relies on the quality of data. Inaccurate or incomplete data can lead to skewed predictions and poor decision-making. Ensuring data cleanliness is crucial for effective predictive analytics.
Data History
Limited historical data can restrict the accuracy of predictions. Longer data histories provide a more comprehensive understanding of trends and patterns, leading to more accurate forecasts.
Privacy Rules
Regulations such as GDPR and CCPA limit the amount of data that can be collected and used for predictive analytics. Businesses must navigate these regulations carefully to ensure compliance while maximizing the utility of their data.
Attribution Mystery
Attributing conversions to specific marketing channels can be challenging. Predictive analytics can struggle to pinpoint which channels are most effective, making it difficult to optimize marketing spend.
Model Bias
Predictive models can inherit biases from the data they are trained on. This can lead to biased predictions, affecting the fairness and accuracy of decisions. Continuous monitoring and adjustment of models are necessary to mitigate bias.
Black Box Models
Some predictive models, especially those based on machine learning, can act as “black boxes” with limited transparency into how predictions are made. This lack of transparency can be a barrier to trust and adoption.
Data Science Expertise Needed
Effective use of predictive analytics requires expertise in data science. Businesses may need to invest in talent or training to fully leverage the power of predictive analytics.
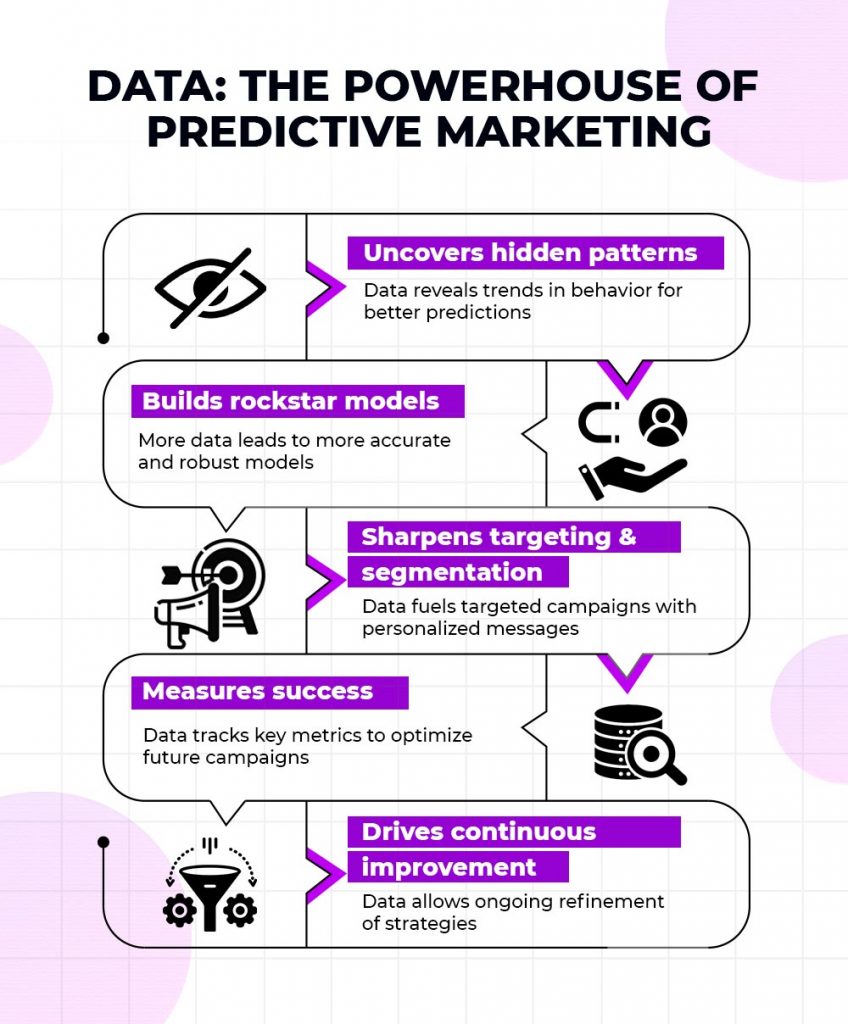
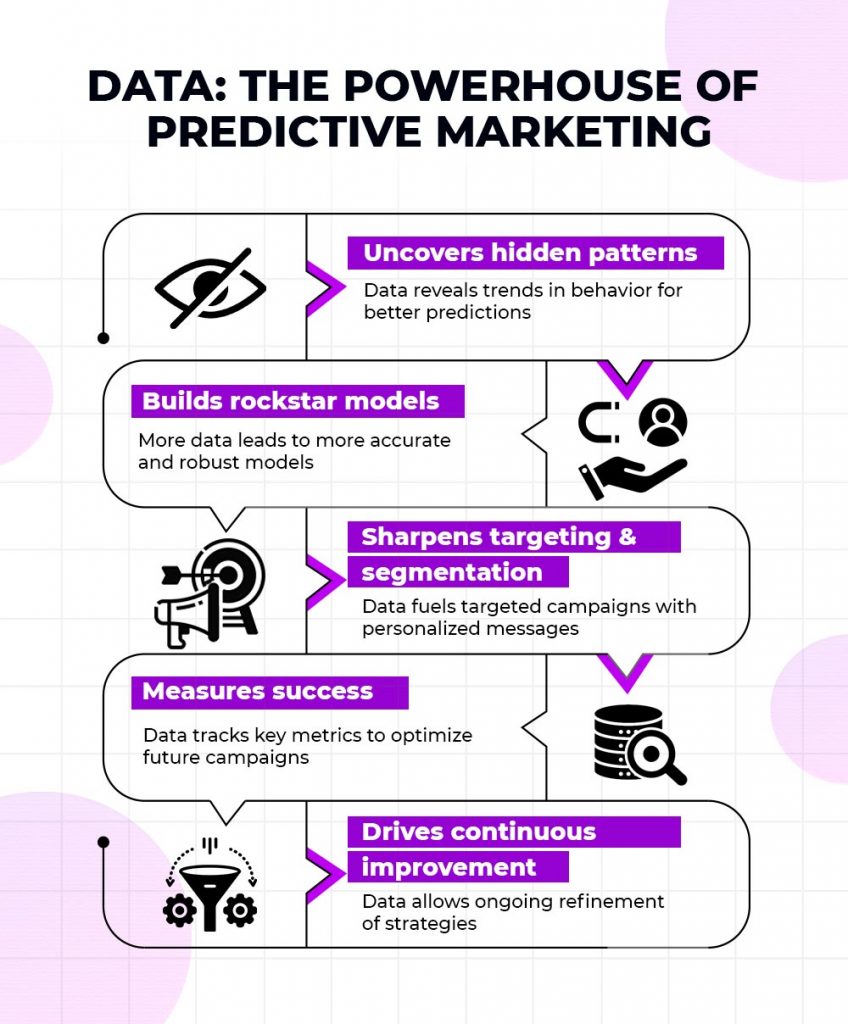
Data: The Powerhouse of Predictive Marketing
Uncovers Hidden Patterns
Data reveals hidden patterns and trends in customer behavior. These insights enable businesses to make more accurate predictions and tailor their strategies accordingly.
Builds Rockstar Models
The more data available, the more accurate and robust predictive models become. Comprehensive datasets allow for the development of models that can handle a wide range of scenarios.
Sharpens Targeting & Segmentation
Data-driven targeting and segmentation allow for highly personalized marketing campaigns. By delivering the right message to the right audience, businesses can significantly improve campaign effectiveness.
Measures Success
Data tracks key performance metrics, providing a clear picture of what works and what doesn’t. This measurement is essential for optimizing future campaigns and achieving continuous improvement.
Drives Continuous Improvement
Predictive analytics fosters a culture of continuous improvement. By regularly analyzing data and refining strategies, businesses can stay ahead of the competition and adapt to changing market conditions.
Deep Dive into Specific Predictive Analytics Techniques
Time Series Analysis
Time series analysis is a powerful technique used to forecast website traffic, sales, and other time-dependent metrics. By examining patterns over time, businesses can anticipate future trends and prepare accordingly. For instance, seasonal trends in website traffic can be identified and leveraged to optimize marketing campaigns.
Machine Learning
Machine learning algorithms play a crucial role in predictive modeling. Techniques such as regression, decision trees, and neural networks can analyze vast amounts of data to make accurate predictions. For example, regression models can predict future sales based on historical data, while decision trees can classify customer segments for targeted marketing.
Customer Lifetime Value (CLTV) Prediction
Predicting Customer Lifetime Value (CLTV) is essential for informed customer segmentation, retention strategies, and marketing investments. By understanding the long-term value of customers, businesses can allocate resources more effectively, focusing on high-value customers and designing strategies to enhance their loyalty. Predictive Marketing Analytics is projected to reach $35.45 billion by 2027 – Allied Market Research
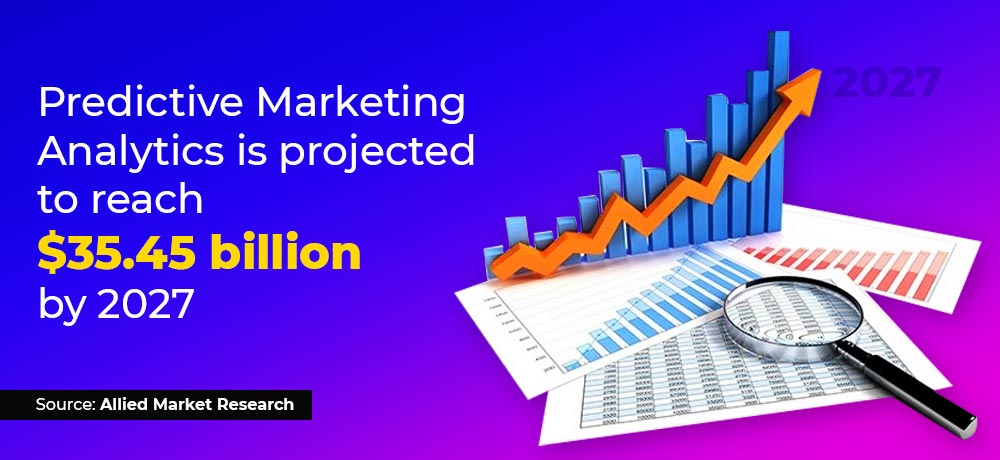
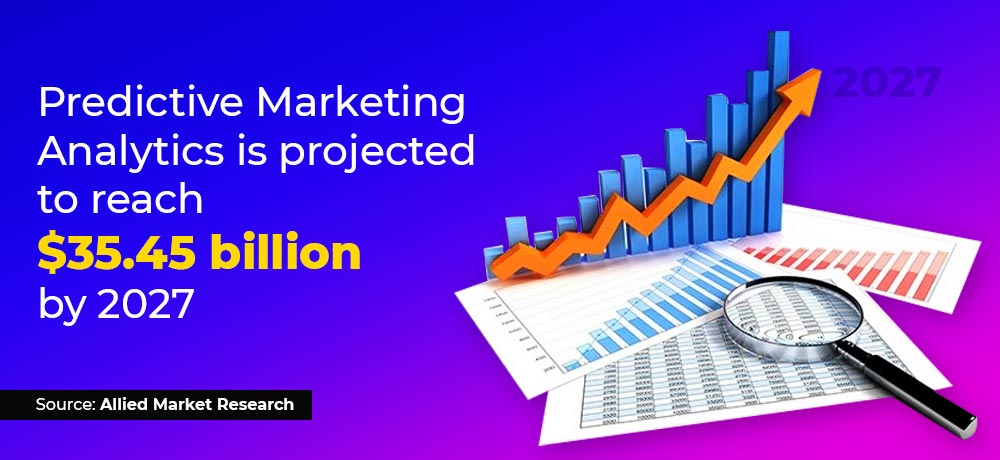
Practical Implementation and Case Studies
Data Preparation
Data preparation is a critical step in predictive analytics. This involves cleaning, transforming, and engineering features to ensure that the data is accurate and suitable for modeling. For example, removing duplicates, filling missing values, and creating new variables can significantly enhance the quality of predictions.
Model Selection and Evaluation
Selecting the appropriate predictive model is essential for accurate predictions. Techniques such as cross-validation and performance metrics (e.g., accuracy, precision, recall) are used to evaluate model performance. For instance, a retail company might use cross-validation to compare different models and choose the one that best predicts sales.
Real-World Examples
Showcasing successful case studies can illustrate the tangible benefits of predictive analytics. For example, an e-commerce company that used predictive analytics to optimize inventory management and reduce stockouts, resulting in increased customer satisfaction and sales.
Addressing Emerging Trends and Challenges
Artificial Intelligence (AI)
The intersection of AI and predictive analytics offers exciting possibilities. Techniques such as natural language processing (NLP) and image recognition can enhance predictive models. For instance, NLP can be used to analyze customer reviews and predict sentiment trends, while image recognition can optimize visual search functions.
Privacy and Ethics
The ethical implications of using predictive analytics cannot be overlooked. Businesses must ensure compliance with data privacy regulations and adopt ethical practices. For example, anonymizing data and obtaining explicit consent from users can help address privacy concerns.
Explainable AI
Understanding complex predictive models is a challenge. Explainable AI (XAI) aims to make these models more interpretable, allowing businesses to understand how predictions are made. This transparency is crucial for building trust and ensuring ethical use of predictive analytics.
Also Read: Advanced Segmentation Techniques in Google Analytics
Actionable Takeaways and Next Steps for predictive analytics with Google Analytics data
Provide Practical Tips
Offer actionable advice for readers on how to get started with predictive analytics. For example, start by identifying key business goals, gathering relevant data, and using simple predictive models to gain initial insights.
Encourage Experimentation
Suggest A/B testing and iterative improvement of predictive models. Experimentation allows businesses to fine-tune their models and strategies, ensuring continuous improvement and better results.
Promote Continuous Learning
Highlight the importance of staying updated on the latest trends and technologies in the field. Encourage readers to participate in workshops, webinars, and online courses to enhance their skills and knowledge.
Potential Additional Sections
Predictive Analytics Tools and Platforms
Provide an overview of popular tools and platforms for implementing predictive analytics. Tools like Google Analytics, Tableau, and Python libraries (e.g., scikit-learn, TensorFlow) can empower businesses to leverage predictive analytics effectively.
Integration with Other Marketing Tools
Discuss how predictive analytics can be integrated with CRM, email marketing, and social media platforms. For example, integrating predictive models with CRM systems can enhance customer segmentation and targeted marketing efforts.
Measuring ROI
Explain how to quantify the impact of predictive analytics on business outcomes. Metrics such as increased sales, improved conversion rates, and reduced customer churn can be used to measure ROI and demonstrate the value of predictive analytics.
Conclusion
Predictive analytics with Google Analytics data offers a wealth of opportunities for businesses to optimize their operations and drive growth. While challenges exist, the benefits far outweigh them. By leveraging data effectively, businesses can make informed decisions, personalize customer experiences, and achieve better results from their marketing efforts. Embracing predictive analytics is not just a trend but a necessity in today’s data-driven world.